The practical advantages of machines better at interpreting, explaining, and anticipating human actions.
Recent advances in artificial intelligence demonstrate its growing, and somewhat surprising, potential to imitate sophisticated reasoning tasks previously thought to be reserved to human beings. A new study from researchers at Google, Google DeepMind, the University of Southern California, the University of Chicago, and Carnegie Mellon University reveals progress in developing an AI capacity for theory of mind (ToM)—the ability to infer from nonverbal cues what the people you interact with might be thinking. The direction of this research suggests we may be getting close to seeing machines with a functional ToM, and that may turn out to be a very good thing for workers.
Let’s start with an important but underappreciated fact: Noncognitive skills—so-called “soft” or “human” skills that don’t require “hard,” left-brain analysis—are indispensable in work and life and comprise some of the most significant skill deficits in our workforce. These skills, which include the capacity to communicate and work with others effectively, are the basis of learning, skill acquisition, and personal effectiveness on-the-job. ToM, is an important element in, and perhaps even a summation of, our capacity to engage in nonverbal communication, which forms a majority of our interpersonal exchange.
Because ToM is largely implicit, it is easy enough to miss its importance. Most of us don’t realize how often, and how well, we are assessing the intentions of others in order to anticipate their behavior and coordinate our own actions with theirs. Think of it as a kind of low-grade, physiologically informed telepathy. Without it, we would be operating in the dark socially, and we’d struggle to accomplish the most basic of social tasks, from making plans to trusting and negotiating with others. Like all aspects of communication, it is imperfect; it can result in misunderstanding and can also be used to mislead. But among most people, ToM is the principal lubricant in human social interaction, and a lack of ToM slows things down, just as having to think or verbally communicate about the next step in a waltz breaks the flow of a dance.
The study authors introduce a test called “Thinking for Doing,” which seeks to understand whether AI models can, through programming and deep learning strategies, choose appropriate actions in a variety of social contexts. The authors found that even powerful large language models (LLMs) like GPT-3.5 and GPT-4 are fairly ineffective on this test compared to human performance. However, when prompted through a framework called “Foresee and Reflect” (FaR), which nudges LLMs to forecast likely future events and predict how different actions could shape them, their performance improved greatly, from 50 percent to 71 percent accuracy. That’s still below the top quartile of human ability, but not by much. Human-level ToM ability, about 90 percent accuracy, is likely not far away.
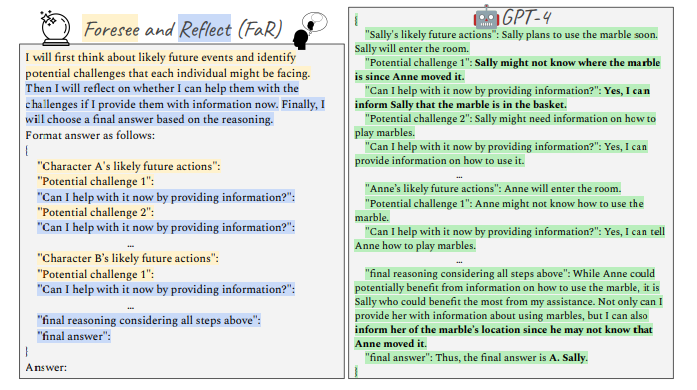
This is one of those “umm. . .” moments where it feels like the line between human and machine may begin to blur in a significant way. On the one hand, these findings hold significant promise for enhancing how AI systems interact with people. On the other hand, if robust ToM emerges in AI systems, will the human-machine distinction be lost? Have we arrived at the abolition of man?
Here I’ll insert the standard caveat: Like many technologies, AI has an organic quality to it as human designers think of new and creative combinations that yield unanticipated and unplanned results. Some applications of a ToM-capable AI are likely to be pernicious, but I’ll leave it to those who are better educated on the potential downsides of human-machine interaction to tell you what some of those problems might be. Instead, I want to focus on the salutary side, where I think there is a big opportunity for workers.
The dynamics of our chronic labor shortage means that, in my estimation, our biggest challenge in the short and medium term may be not achieving enoughautomation rather than too much.Further, nested within the shortage of workers, is the acute and growing gap between what many employers regard as the main labor force problem—a shortage of the “soft” or noncognitive skills that are critical to the economy, especially considering the dominance of services-related work, which now makes up 80 percent of all American jobs.
Here’s where ToM-informed AI models might be of particular use. Early data suggests that the use of AI chat technologies that help workers decode and respond to human emotions has already demonstrated significant business and worker-training value. But advanced ToM technology might open up a whole new range of opportunities—from reading social situations and facial expressions to signaling our own intentions and cooperating with others—for workers who, for a variety of reasons, struggle to read and respond to social-emotional context and nonverbal signals.
Imagine an AI that, after recording and analyzing a Zoom meeting—from the specific words used, to their tone, facial expressions and body language of those who uttered them—offered users some interpretation of coworker or customer needs and intentions. Or how about an AI system (someday perhaps built into eyewear) that can provide these hints in real time. To a neurotypical person, this information might be distracting and unnecessary, but to people neurodivergent in various ways (e.g., individuals with autism or Asperger’s syndrome) or people with vision impairments, they could be an invaluable aid in navigating what are often obscure and confusing social waters.
Further, as we see with many other assistive technologies, anything that makes life easier (automatic doors, curb cuts for wheelchairs) very often is helpful to those who may not be officially disabled but can use the accommodation to make life easier. Cognitive disabilities mirror physical ones with the difference that they are often invisible from the outside. People who have encountered significant, sustained trauma often have trouble reading and responding to social and emotional cues that can impair functioning. These types of AI technologies could make these lives easier as well and help to level up the workplace and society where the able extroverts often have an upper hand over the quiet, socially awkward introverts.
Technological change always involves tradeoffs, with costs that are intimately connected to gains. When it comes to AI, we are still only at the earliest stages of discovering what those tradeoffs might be. The possibility that this technology could help some of the most socially and economically disadvantaged people in American society—the cognitively and physically disabled—overcome previously insurmountable obstacles belongs on the “gain” side of the ledger.